4 k* x5 R# T3 [! e+ T
点击蓝字
8 L4 Y" e8 E5 }' O2 K 关注我们 / X+ T2 Y' I4 N. G% H
文章发表于 Ocean and Coastal Management 242 (2023) 106679
( m4 g; G u* i 文章链接:
" v5 A+ k# ~3 q6 g0 _ https://linkinghub.elsevier.com/retrieve/pii/S0964569123002041 1 J8 v3 }6 ~2 y/ k* m) h. n
文章标题: Knowledge graph for maritime pollution regulations based on deep learning methods
/ M' L. \$ \3 O* F2 U* l 文章导读
: ?6 g2 D. Y" r$ h 随着全球贸易需求的不断增长,航运活动比以往任何时候都更加活跃,这对全球海洋安全和污染预防构成了巨大挑战。船舶污染防治和海洋资源的可持续发展一直是今后研究的重点。港口国政府根据国际组织和政府制定的规定,完成了多次港口国管制(PSC)检查,以应对来自船舶的污染预防挑战。从中国海事委员会官员的角度来看,研究重点是规定在现场检查中的方便适用性。
# r* d; x, f: S1 F$ ] 本文致力于实施一种智能的方法来管理污染预防法规和支持检查。开发了一个深度学习知识提取模型,即变压器-多卷积神经网络模型(BERT-MCNN)的双向编码器表示,从中国海洋污染预防相关的法律、法规、规则和惯例中提取一线管理所需的信息。
. [( L0 @$ ?' n5 T2 M) R* h 文章摘要 - f$ B) v, w% R
航运业的污染预防已经引起了全球政府主管部门的关注。细致的港国管制(PSC)检查是防止船舶污染的有效途径。PSC检查涉及各种法规,但目前缺乏有效的方法来利用这些法规来支持在前线迅速作出海洋污染预防管理的有效决策。本研究开发了一个深度学习知识提取模型,即变压器-多卷积神经网络模型(BERT-MCNN)的双向编码器表示,从中国海洋污染预防相关的法律、法规、规则和惯例中提取一线管理所需的信息。结果表明,基于深度学习方法可以形成海洋污染预防调控知识图,多关系提取和命名实体识别的准确率分别为92.4%和92.7%。基于图数据库Neo4j,应用提取结果形成的知识图,可以有效地提供知识查询,并协助PSC人员在检查现场进行决策。本研究提出的方法可用于我国污染预防法规的分析,支持污染预防检查。
2 _0 q4 P: R: d2 s7 ~ 原文信息 2 N I5 ~1 |' ^* G0 ~8 W$ s: w2 ?; O
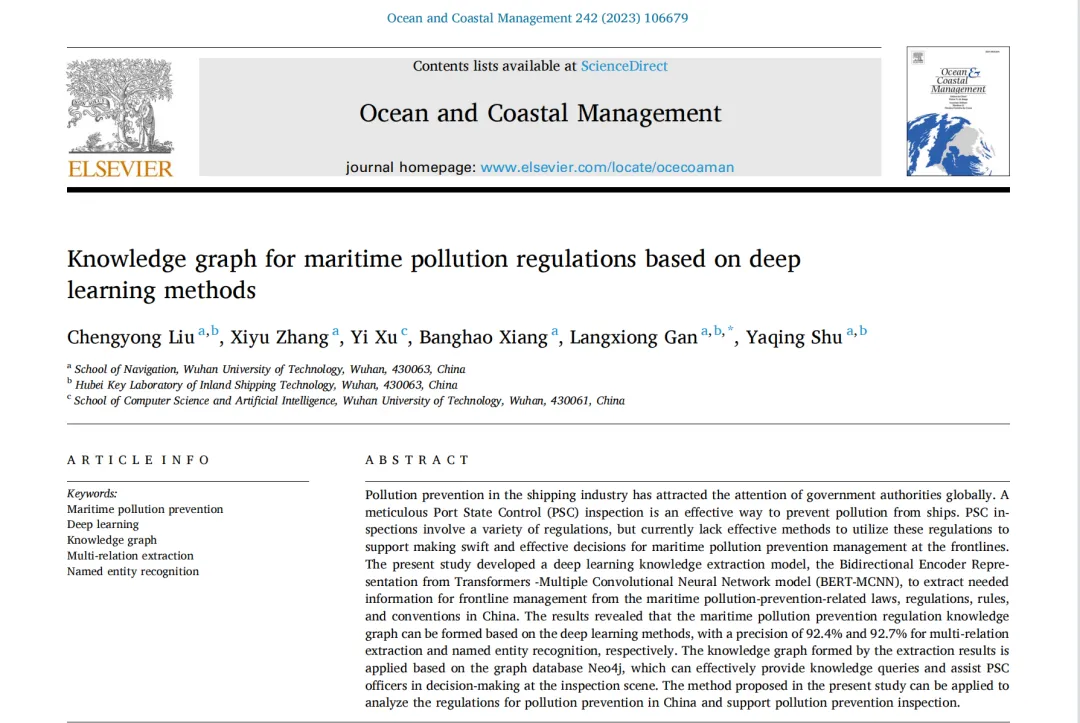
8 d" U) Z* d3 m( c' e) ` 图1 图形摘要 ; c' }6 C% Q7 T# ~, i, [
图2 实体-关系三元组的整体组合图 & W; h8 O9 @& U0 A' z
图3 BERT-MCNN模型的体系结构和嵌入层的细节 5 C* D6 X( [5 g% l& O1 S
图4 PCNN和VCNN模块的详细架构(A部分为PCNN模块,B部分为VCNN模块 # Q+ u2 w9 }& w* k: y, N
图5 在Neo4j中存储一个三重的结果(部分)
+ G- W$ r8 o; B' \ 图6 石油记录册的子图(第二部分) 6 ]1 h9 ?* T q% Q* |
图7 油清单和油子图
) ^" H' x: Q. a: ~# y 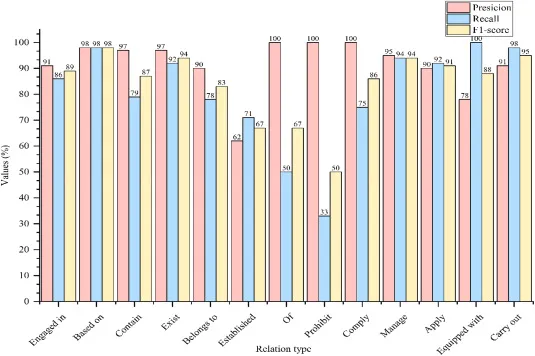 ) x5 ^: f$ _" O3 A9 A2 A2 K$ O
图8在测试数据集中的每个关系的值
- z6 w0 ~2 R$ d5 H, a 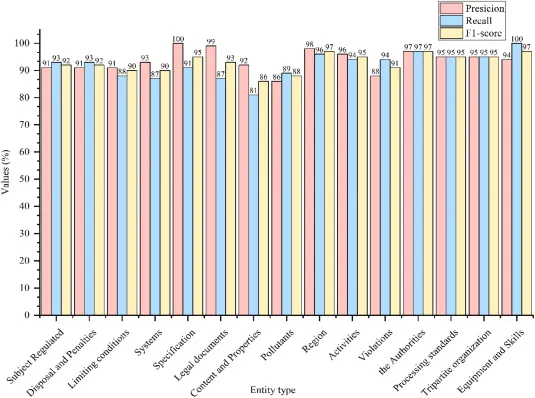 j- D4 q1 r. X2 J% E
图9测试数据集中的每个图元的值 ; o. U! g' M% F6 J! G( e9 ]
+ O0 {% Z# ]7 f, `9 P- Z 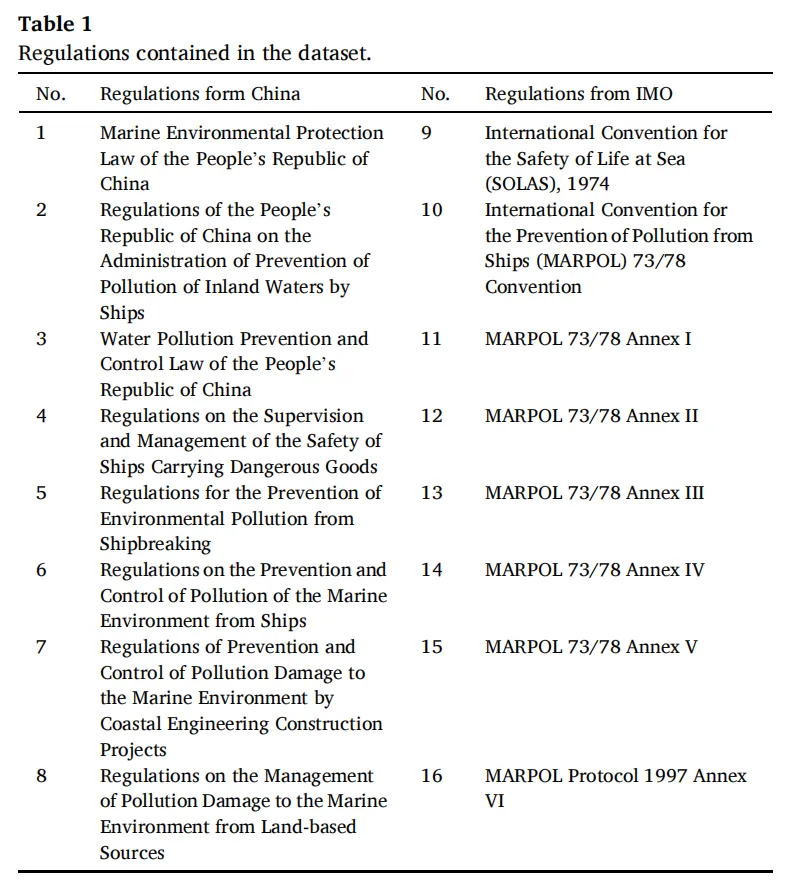 ; y6 b! [5 }# s1 a3 [0 z
表1 数据集中包含的规则
8 Z0 o1 b- f$ p# Z0 f l1 K. k 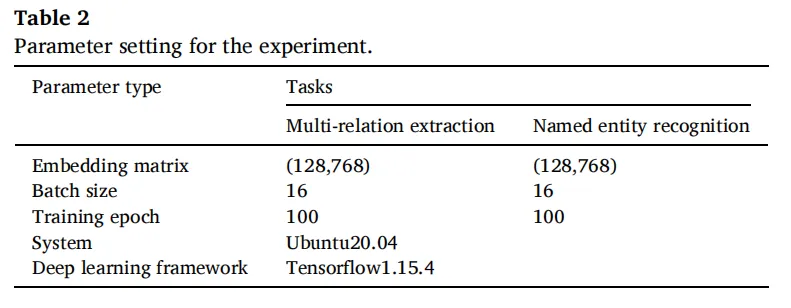
' r# z/ }2 n1 e* ?" B 表2 本实验的参数设置 : I5 b7 p" H3 ]& ]9 @5 ~7 b
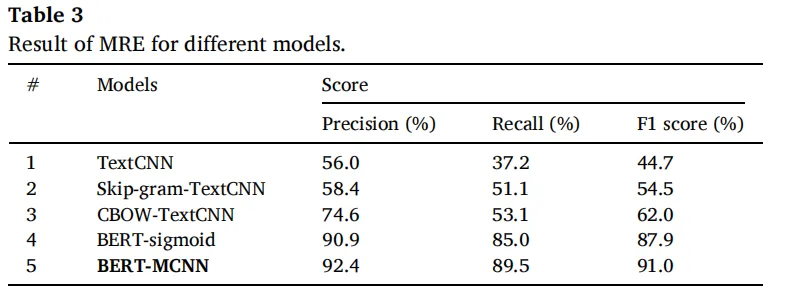 % m0 x- [6 Y) I, l# w
表3 对不同模型的MRE的结果 2 h& ]0 `7 _6 O3 \5 b5 _% b" e# S
7 h* U# i; a, w" U, j 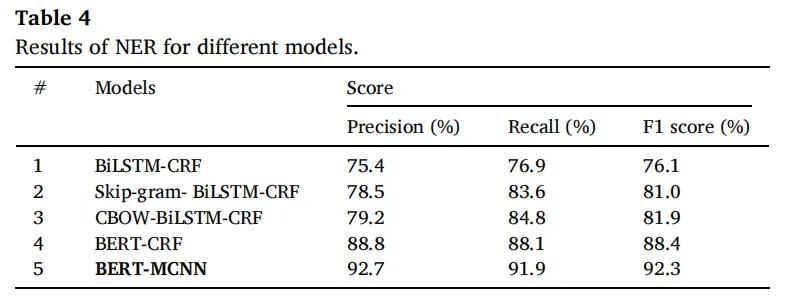
" n( w: a: z" m7 r' J: D/ G% K 表4 不同模型的NER结果
- i" R! b$ j3 K8 W5 c 4 c8 ~9 \) e2 P* S
ABSTRACT Pollution prevention in the shipping industry has attracted the attention of government authorities globally. Ameticulous Port State Control (PSC) inspection is an effective way to prevent pollution from ships. PSC in spections involve a variety of regulations, but currently lack effective methods to utilize these regulations to support making swift and effective decisions for maritime pollution prevention management at the frontlines.The present study developed a deep learning knowledge extraction model, the Bidirectional Encoder Representation from Transformers -Multiple Convolutional Neural Network model (BERT-MCNN), to extract needed information for frontline management from the maritime pollution-prevention-related laws, regulations, rules, and conventions in China. The results revealed that the maritime pollution prevention regulation knowledge graph can be formed based on the deep learning methods, with a precision of 92.4% and 92.7% for multi-relation extraction and named entity recognition, respectively. The knowledge graph formed by the extraction results is applied based on the graph database Neo4j, which can effectively provide knowledge queries and assist PSC officers in decision-making at the inspection scene. The method proposed in the present study can be applied to analyze the regulations for pollution prevention in China and support pollution prevention inspection.- v( w: }% U; O; ?( h1 S
KEYWORDS Maritime pollution preventionDeep learningKnowledge graphMulti-relation extractionNamed entity recognition% U0 }3 _1 J9 I# g/ ?
推荐阅读
V" m F% {. Q) E0 Y$ U& U2 s, C, u 期刊主页
% g! n) C6 w5 l. s' [ OCMA期刊编辑专家团队 - L8 m. J% Q% j$ e
官宣!Ocean & Coastal Management 最新Aims & Scope 7 x& k8 @6 R9 f0 J
关注!Ocean & Coastal Management 是SCI /SSCI双检索期刊 : t1 n- J y1 v8 y. E+ c/ k
Call for Papers on the Special Issue in RSMA # N( i: i# t& |! k/ h9 |
本期编辑
( d& T# H8 T" \7 i. u 深圳大学研究生 姚俊屹
" p2 q1 `, U. K( |4 P 研究方向:国际海事发展治理
# c1 \7 y$ E! @8 k+ L- k4 S# G
! }6 C. U8 ^3 X5 l, Y! G. w
# Q/ m5 |1 S- t |